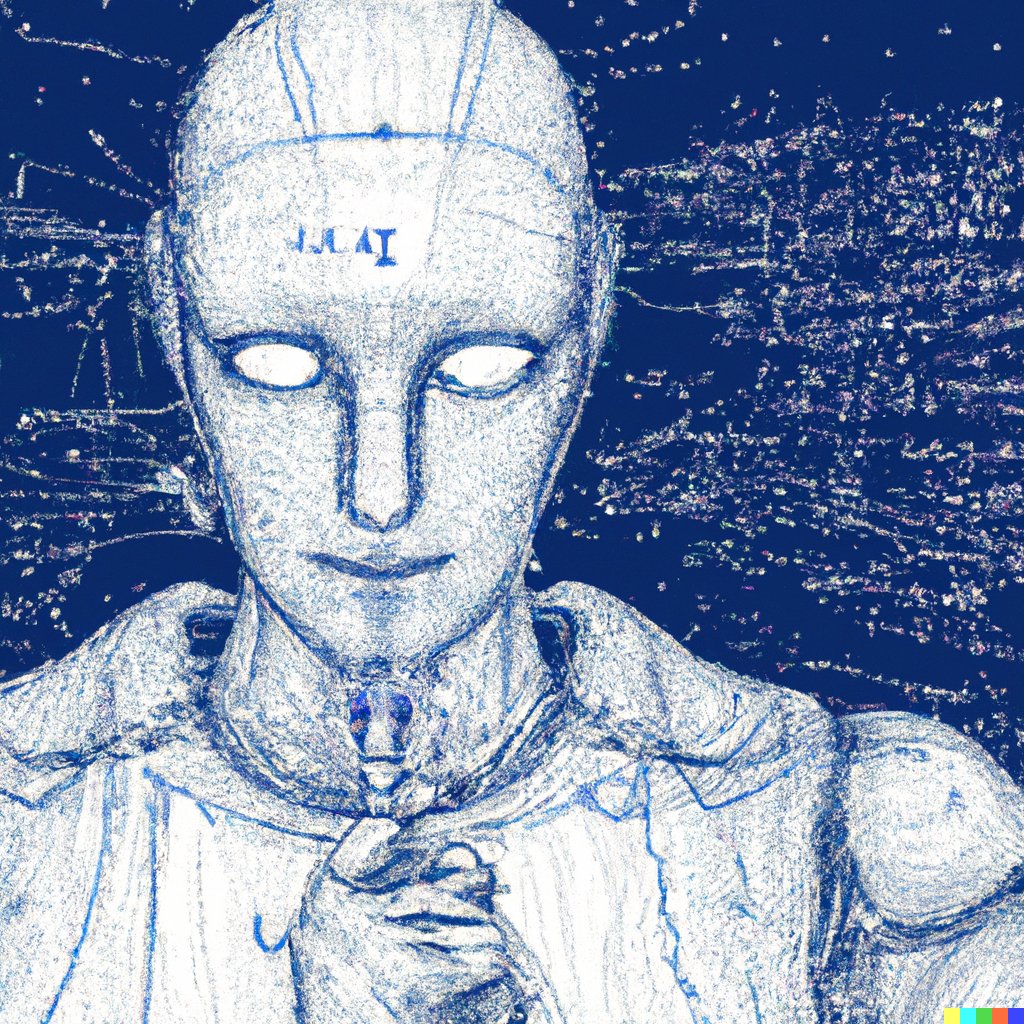
This week has been one for reflection, reconsideration, and yes, even a bit of re-routing on our journey into AI in pharma. Although I underwent cataract surgery, which understandably slowed things down, it has been a period of learning and adapting. Today, I will share my recent experiences and offer some valuable insights that can help you better understand the potential and challenges of integrating clinical artificial intelligence into medical writing and pharmaceutical medical information.
Progress and Ponderings
It started off well. I believed I had made significant strides in text extraction from a PDF clinical trial, cleanly separating the content by paragraphs. The process appeared to be promising, a big first step towards AI in medical writing and research. But, as we delve deeper into the world of machine learning in pharma, we continually discover new considerations and challenges.
In reflection, I realized that it’s not merely about extracting text and summarizing it; it’s about creating a tool that’s effective and user-friendly for our peers in the pharmaceutical industry. I envision an interface where users can select what they want to include in the summary. The extracted text would be displayed, divided into sections, each section accompanied by a checkbox. Users could select the sections they want to summarize with a simple click, then hit a “summarize” button to generate a concise summary of the selected content.
The proposed sections would ideally include:
- Objective
- Design summary (RCT, prospective, retrospective, etc.)
- Eligibility Criteria
- Statistics (power, alpha, etc.)
- Treatment groups and treatments
- Endpoints (primary, secondary, safety, description and results)
- Study limitations
- Author’s conclusions
In the long term, the program should enable users to select a key endpoint for their standard response, automatically placing a summary sentence at the beginning.
Revisiting My Approach
Given this new direction, I plan to explore two different approaches to achieve this goal. One approach is to engineer a software solution that can efficiently extract the relevant information for each of the above sections from the paragraphs of text. The second approach is to concurrently build a machine learning model capable of identifying these sections. This two-pronged strategy will allow me to compare and identify the most effective method.
However, as we know, every journey encounters a few bumps. One of the roadblocks I encountered this week was the quality of PDF extraction. It turns out that the extraction is not as clean as I initially thought, leading to the need for further refinement.
Embracing the Learning Curve
Even though this week didn’t produce the rapid progress I had hoped for, it served as a stark reminder that every step in this journey, no matter how small, holds its value. The process of trial, error, and reassessment is invaluable, offering critical insights that contribute to the refinement and success of this endeavor into AI in pharma med info. And though it was a slower week, my vision—both literally and figuratively—has become clearer post-surgery.
In conclusion, the path to incorporating clinical artificial intelligence into medical writing in med info is a winding one, filled with learning opportunities at every turn. But it is through these trials and tribulations that we make progress and find innovative ways to enhance medical affairs and medical information in the pharmaceutical industry. As we continue on this journey, we remain committed to sharing our experiences, offering insights, and providing resources to support your exploration of AI in pharma. Together, we’ll navigate this exciting landscape, bringing the future of pharmaceutical research into the present.
Remember, the greatest value lies in the journey itself and the learnings we acquire along the way. Here’s to clearer vision and further progress in the coming week!