Harnessing the Power of AI in Pharma: My Journey Towards Self-Trained Trial Summarization
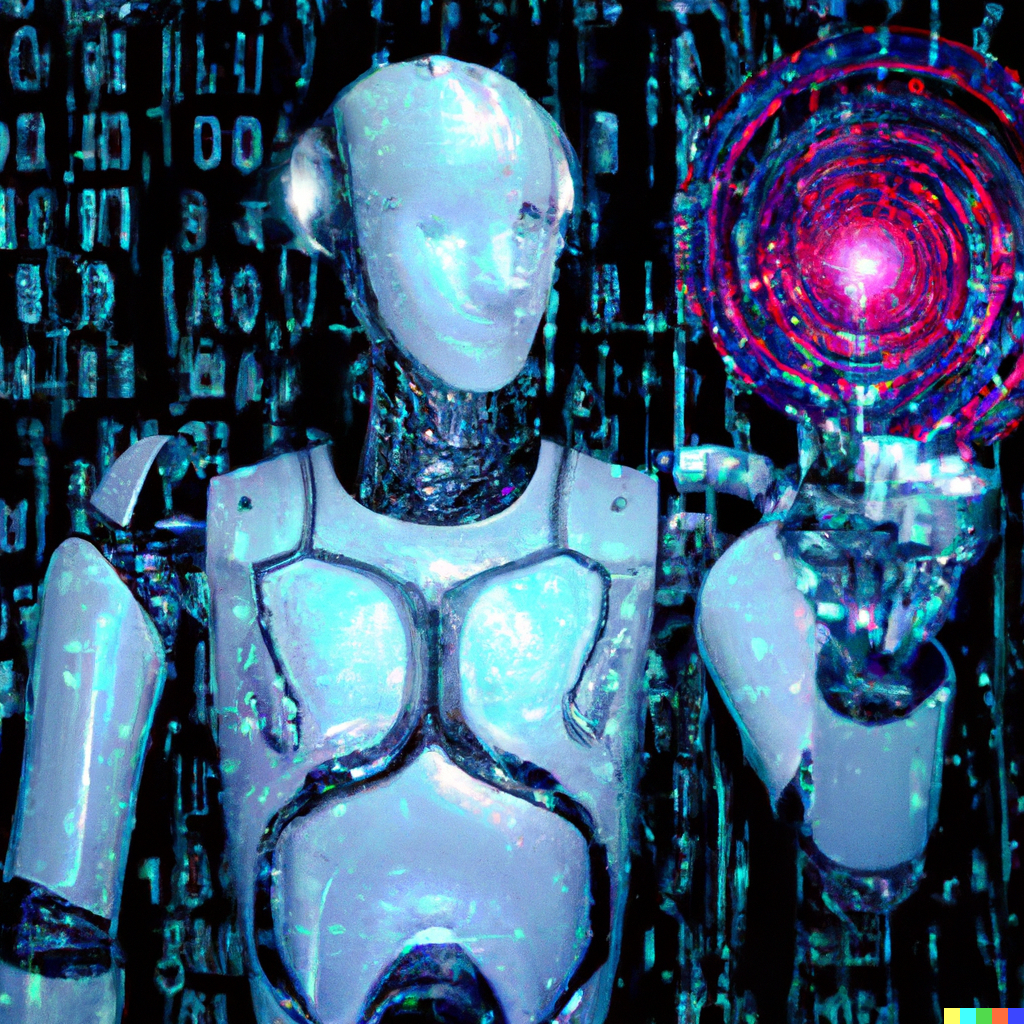
Over the past week, I embarked on a fascinating journey, combining my passions for pharmaceutical research, medical writing, and innovative technology. As a healthcare professional consistently engaging with the complexities of the American Medical Association’s 11th Edition style, I sought to leverage machine learning to streamline and personalize my work in the form of a tailor-made model. With the promising potential of AI in pharma, I embarked on this path to create a solution that could synthesize and summarize data, the way I envisioned.
As the first step in this venture, I focused on developing a unique dataset to train my machine learning model. The traditional approach would be to manually create hundreds of summaries, a time-consuming and tedious process. Instead, I decided to automate this using an AI model to generate the summaries. This ambitious undertaking was driven by my keen interest in clinical artificial intelligence and its transformative potential in pharma.
To facilitate this, I constructed a program designed to summarize based on pre-existing data, primarily drawing information from PDFs of clinical trials. This, however, wasn’t without its challenges. Initially, I encountered difficulties in extracting text from PDFs in a clean and useful manner, a hurdle which seemed daunting at first.
I attempted numerous approaches to address this obstacle, only to find a promising method in an online example. Adapting and optimizing this method to meet my needs, I successfully engineered a program capable of summarizing PDF clinical trials effectively. The resulting summaries were satisfactory, getting me around 75% of the way to my goal.

With these preliminary summaries in hand, my next step involved refining these outputs. This required meticulous editing to ensure they were perfect representations of the style I aimed for my model to emulate. My plan was to start with an initial set of 10 refined summaries, which would serve as the foundational data to train my model.
The subsequent phase involved using the trained model to generate the next batch of 10 summaries. Each of these would then be edited to perfection, expanding the dataset to 20. This iterative process of generating, editing, and expanding was repeated, with each cycle bringing me closer to a model that could produce summaries aligning closely with my desired style.
This method, while admittedly tedious, held the promise of a significant payoff. The goal was not just to create a model that could generate summaries but one that could replicate my personal style, resonating with the strict standards of AMA 11th edition style while maintaining a succinct and clear summary. This approach embodied the immense potential of machine learning in pharma and the personalization it can offer.
As I delve deeper into this endeavor, I remain optimistic about the potential outcome. The path towards integrating AI in pharma and particularly in clinical trial summarization is a promising one, albeit marked with challenges and learning curves. I invite you all to follow my journey as I navigate these exciting waters, demonstrating the potential of machine learning in pharma and clinical artificial intelligence.
Please do not hesitate to get in touch if you are interested in learning more about this project, have ideas to share, or simply wish to connect. The fusion of healthcare, pharmaceuticals, and AI is a vast landscape, and together we can explore and shape its potential.
Stay tuned as I continue to share more about this innovative journey and the transformative role of AI in pharma.